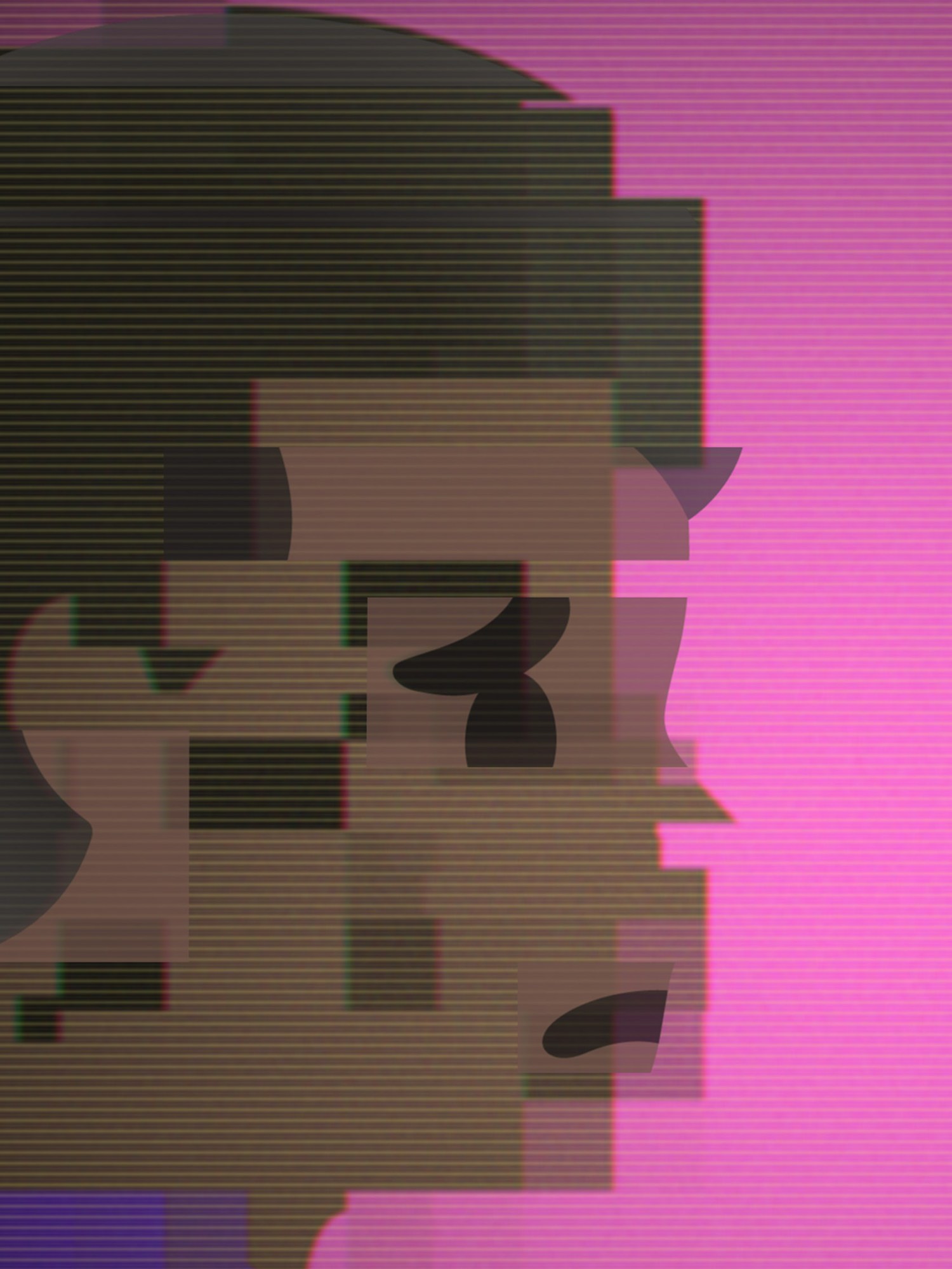
It was a stranger who first brought home for me how big this year’s vibe shift was going to be. As we waited for a stuck elevator together in March, she told me she had just used ChatGPT to help her write a report for her marketing job. She hated writing reports because she didn’t think she was very good at it. But this time her manager had praised her. Did it feel like cheating? Hell no, she said. You do what you can to keep up.
That stranger’s experience of generative AI is one among millions. People in the street (and in elevators) are now figuring out what this radical new technology is for and wondering what it can do for them. In many ways the buzz around generative AI right now recalls the early days of the internet: there’s a sense of excitement and expectancy—and a feeling that we’re making it up as we go.
That is to say, we’re in the dot-com boom, circa 2000. Many companies will go bust. It may take years before we see this era’s Facebook (now Meta), Twitter (now X), or TikTok emerge. “People are reluctant to imagine what could be the future in 10 years, because no one wants to look foolish,” says Alison Smith, head of generative AI at Booz Allen Hamilton, a technology consulting firm. “But I think it’s going to be something wildly beyond our expectations.”
Here’s the catch: it is impossible to know all the ways a technology will be misused until it is used.
The internet changed everything—how we work and play, how we spend time with friends and family, how we learn, how we consume, how we fall in love, and so much more. But it also brought us cyber-bullying, revenge porn, and troll factories. It facilitated genocide, fueled mental-health crises, and made surveillance capitalism—with its addictive algorithms and predatory advertising—the dominant market force of our time. These downsides became clear only when people started using it in vast numbers and killer apps like social media arrived.
Generative AI is likely to be the same. With the infrastructure in place—the base generative models from OpenAI, Google, Meta, and a handful of others—people other than the ones who built it will start using and misusing it in ways its makers never dreamed of. “We’re not going to fully understand the potential and the risks without having individual users really play around with it,” says Smith.
Generative AI was trained on the internet and so has inherited many of its unsolved issues, including those related to bias, misinformation, copyright infringement, human rights abuses, and all-round economic upheaval. But we’re not going in blind.
Here are six unresolved questions to bear in mind as we watch the generative-AI revolution unfold. This time around, we have a chance to do better.
1
Will we ever mitigate the bias problem?
Bias has become a byword for AI-related harms, for good reason. Real-world data, especially text and images scraped from the internet, is riddled with it, from gender stereotypes to racial discrimination. Models trained on that data encode those biases and then reinforce them wherever they are used.
Chatbots and image generators tend to portray engineers as white and male and nurses as white and female. Black people risk being misidentified by police departments’ facial recognition programs, leading to wrongful arrest. Hiring algorithms favor men over women, entrenching a bias they were sometimes brought in to address.
Without new data sets or a new way to train models (both of which could take years of work), the root cause of the bias problem is here to stay. But that hasn’t stopped it from being a hot topic of research. OpenAI has worked to make its large language models less biased using techniques such as reinforcement learning from human feedback (RLHF). This steers the output of a model toward the kind of text that human testers say they prefer.
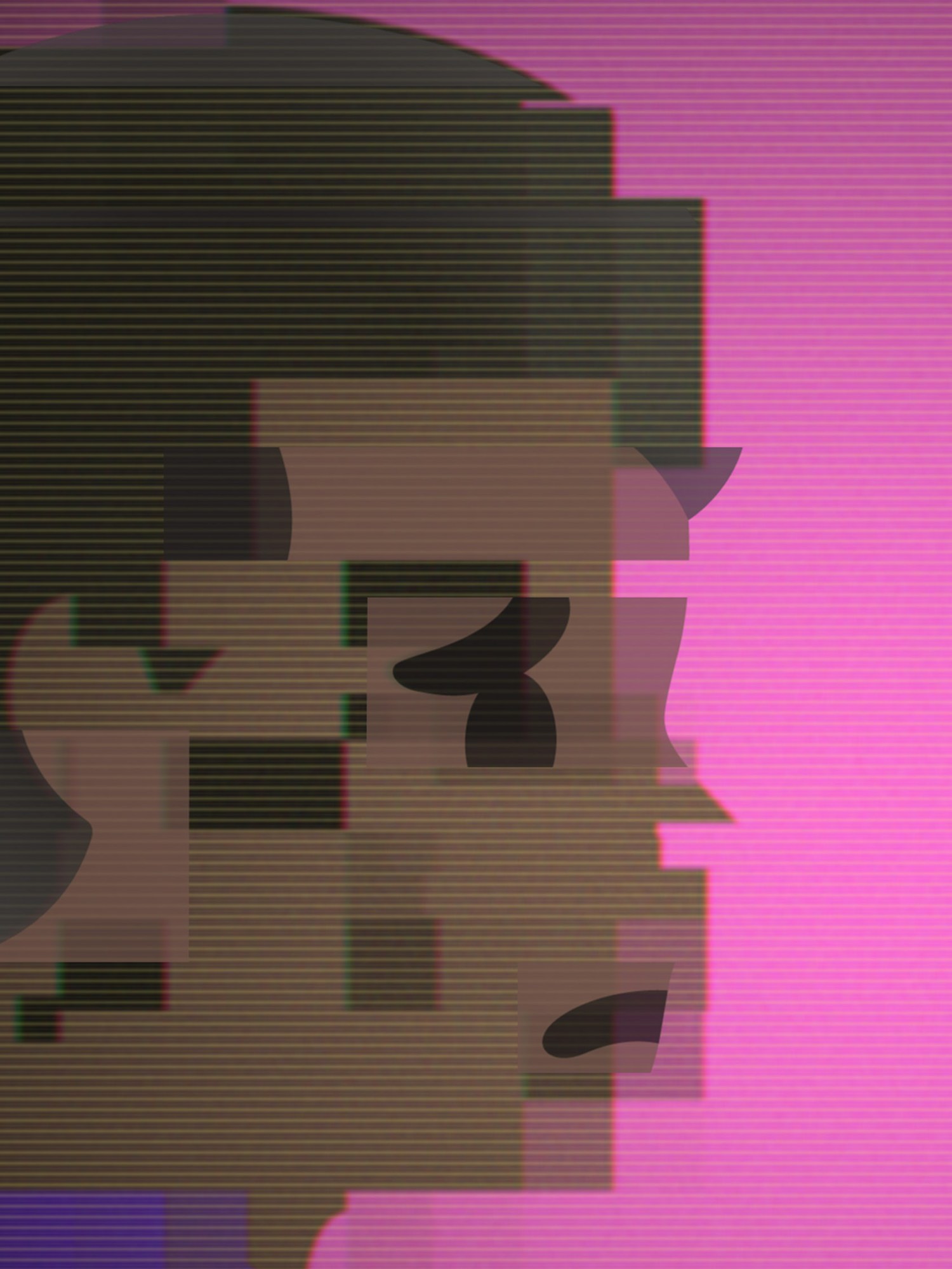
Other techniques involve using synthetic data sets. For example, Runway, a startup that makes generative models for video production, has trained a version of the popular image-making model Stable Diffusion on synthetic data such as AI-generated images of people who vary in ethnicity, gender, profession, and age. The company reports that models trained on this data set generate more images of people with darker skin and more images of women. Request an image of a businessperson, and outputs now include women in headscarves; images of doctors will depict people who are diverse in skin color and gender; and so on.
Critics dismiss these solutions as Band-Aids on broken base models, hiding rather than fixing the problem. But Geoff Schaefer, a colleague of Smith’s at Booz Allen Hamilton who is head of responsible AI at the firm, argues that such algorithmic biases can expose societal biases in a way that’s useful in the long run.
As an example, he notes that even when explicit information about race is removed from a data set, racial bias can still skew data-driven decision-making because race can be inferred from people’s addresses—revealing patterns of segregation and housing discrimination. “We got a bunch of data together in one place, and that correlation became really clear,” he says.
Schaefer thinks something similar could happen with this generation of AI: “These biases across society are going to pop out.” And that will lead to more targeted policymaking, he says.
But many would balk at such optimism. Just because a problem is out in the open doesn’t guarantee it’s going to get fixed. Policymakers are still trying to address social biases that were exposed years ago—in housing, hiring, loans, policing, and more. In the meantime, individuals live with the consequences.
Prediction: Bias will continue to be an inherent feature of most generative AI models. But workarounds and rising awareness could help policymakers address the most obvious examples.
2
How will AI change the way we apply copyright?
Outraged that tech companies should profit from their work without consent, artists and writers (and coders) have launched class action lawsuits against OpenAI, Microsoft, and others, claiming copyright infringement. Getty is suing Stability AI, the firm behind the image maker Stable Diffusion.
These cases are a big deal. Celebrity claimants such as Sarah Silverman and George R.R. Martin have drawn media attention. And the cases are set to rewrite the rules around what does and does not count as fair use of another’s work, at least in the US.
But don’t hold your breath. It will be years before the courts make their final decisions, says Katie Gardner, a partner specializing in intellectual-property licensing at the law firm Gunderson Dettmer, which represents more than 280 AI companies. By that point, she says, “the technology will be so entrenched in the economy that it’s not going to be undone.”
In the meantime, the tech industry is building on these alleged infringements at breakneck pace. “I don’t expect companies will wait and see,” says Gardner. “There may be some legal risks, but there are so many other risks with not keeping up.”
Some companies have taken steps to limit the possibility of infringement. OpenAI and Meta claim to have introduced ways for creators to remove their work from future data sets. OpenAI now prevents users of DALL-E from requesting images in the style of living artists. But, Gardner says, “these are all actions to bolster their arguments in the litigation.”
Google, Microsoft, and OpenAI now offer to protect users of their models from potential legal action. Microsoft’s indemnification policy for its generative coding assistant GitHub Copilot, which is the subject of a class action lawsuit on behalf of software developers whose code it was trained on, would in principle protect those who use it while the courts shake things out. “We’ll take that burden on so the users of our products don’t have to worry about it,” Microsoft CEO Satya Nadella told MIT Technology Review.
At the same time, new kinds of licensing deals are popping up. Shutterstock has signed a six-year deal with OpenAI for the use of its images. And Adobe claims its own image-making model, called Firefly, was trained only on licensed images, images from its Adobe Stock data set, or images no longer under copyright. Some contributors to Adobe Stock, however, say they weren’t consulted and aren’t happy about it.
Resentment is fierce. Now artists are fighting back with technology of their own. One tool, called Nightshade, lets users alter images in ways that are imperceptible to humans but devastating to machine-learning models, making them miscategorize images during training. Expect a big realignment of norms around sharing and repurposing media online.
Prediction: High-profile lawsuits will continue to draw attention, but that’s unlikely to stop companies from building on generative models. New marketplaces will spring up around ethical data sets, and a cat-and-mouse game between companies and creators will develop.
3
How will it change our jobs?
We’ve long heard that AI is coming for our jobs. One difference this time is that white-collar workers—data analysts, doctors, lawyers, and (gulp) journalists—look to be at risk too. Chatbots can ace high school tests, professional medical licensing examinations, and the bar exam. They can summarize meetings and even write basic news articles. What’s left for the rest of us? The truth is far from straightforward.
Many researchers deny that the performance of large language models is evidence of true smarts. But even if it were, there is a lot more to most professional roles than the tasks those models can do.
Last summer, Ethan Mollick, who studies innovation at the Wharton School of the University of Pennsylvania, helped run an experiment with the Boston Consulting Group to look at the impact of ChatGPT on consultants. The team gave hundreds of consultants 18 tasks related to a fictional shoe company, such as “Propose at least 10 ideas for a new shoe targeting an underserved market or sport” and “Segment the footwear industry market based on users.” Some of the group used ChatGPT to help them; some didn’t.
The results were striking: “Consultants using ChatGPT-4 outperformed those who did not, by a lot. On every dimension. Every way we measured performance,” Mollick writes in a blog post about the study.
Many businesses are already using large language models to find and fetch information, says Nathan Benaich, founder of the VC firm Air Street Capital and leader of the team behind the State of AI Report, a comprehensive annual summary of research and industry trends. He finds that welcome: “Hopefully, analysts will just become an AI model,” he says. “This stuff’s mostly a big pain in the ass.”
His point is that handing over grunt work to machines lets people focus on more fulfilling parts of their jobs. The tech also seems to level out skills across a workforce: early studies, like Mollick’s with consultants and others with coders, suggest that less experienced people get a bigger boost from using AI. (There are caveats, though. Mollick found that people who relied too much on GPT-4 got careless and were less likely to catch errors when the model made them.)
Generative AI won’t just change desk jobs. Image- and video-making models could make it possible to produce endless streams of pictures and film without human illustrators, camera operators, or actors. The strikes by writers and actors in the US in 2023 made it clear that this will be a flashpoint for years to come.
Even so, many researchers see this technology as empowering, not replacing, workers overall. Technology has been coming for jobs since the industrial revolution, after all. New jobs get created as old ones die out. “I feel really strongly that it is a net positive,” says Smith.
But change is always painful, and net gains can hide individual losses. Technological upheaval also tends to concentrate wealth and power, fueling inequality.
“In my mind, the question is no longer about whether AI is going to reshape work, but what we want that to mean,” writes Mollick.
Prediction: Fears of mass job losses will prove exaggerated. But generative tools will continue to proliferate in the workplace. Roles may change; new skills may need to be learned.
4
What misinformation will it make possible?
Three of the most viral images of 2023 were photos of the pope wearing a Balenciaga puffy, Donald Trump being wrestled to the ground by cops, and an explosion at the Pentagon. All fake; all seen and shared by millions of people.
Using generative models to create fake text or images is easier than ever. Many warn of a misinformation overload. OpenAI has collaborated on research that highlights many potential misuses of its own tech for fake-news campaigns. In a 2023 report it warned that large language models could be used to produce more persuasive propaganda—harder to detect as such—at massive scales. Experts in the US and the EU are already saying that elections are at risk.
It was no surprise that the Biden administration made labeling and detection of AI-generated content a focus of its executive order on artificial intelligence in October. But the order fell short of legally requiring tool makers to label text or images as the creations of an AI. And the best detection tools don’t yet work well enough to be trusted.
The European Union’s AI Act, agreed this month, goes further. Part of the sweeping legislation requires companies to watermark AI-generated text, images, or video, and to make it clear to people when they are interacting with a chatbot. And the AI Act has teeth: the rules will be binding and come with steep fines for noncompliance.
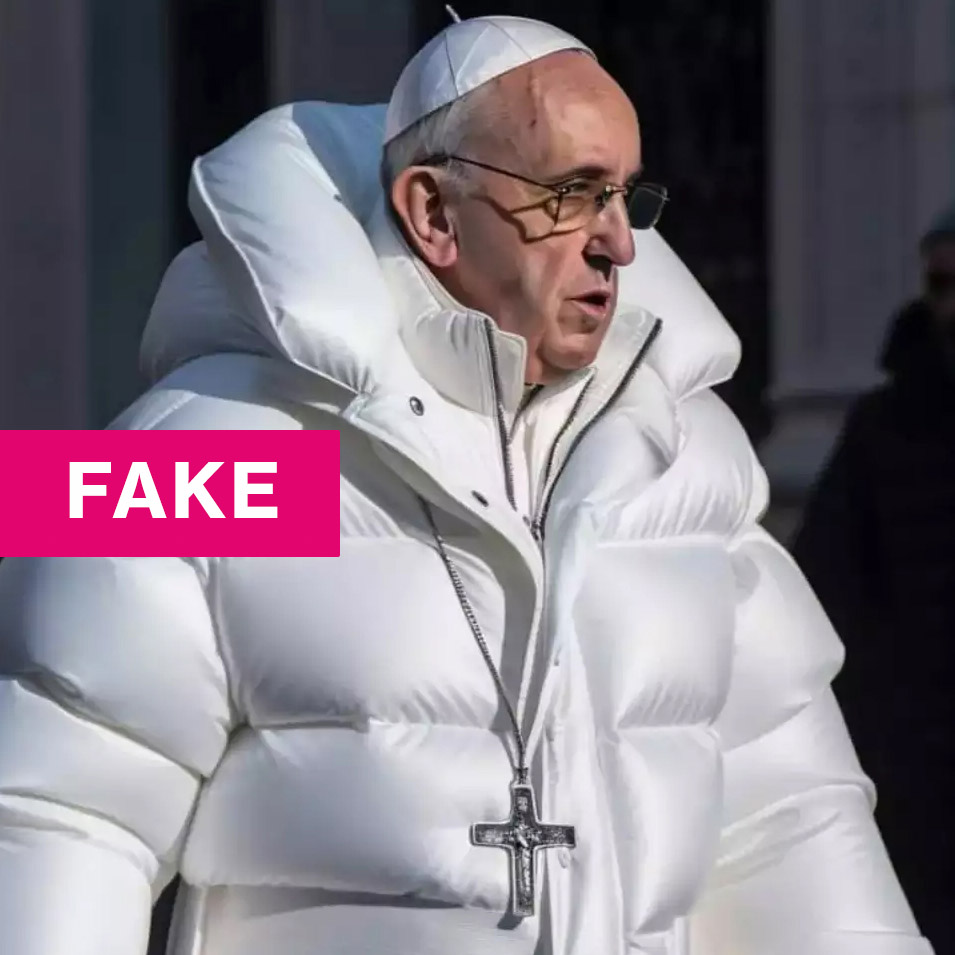
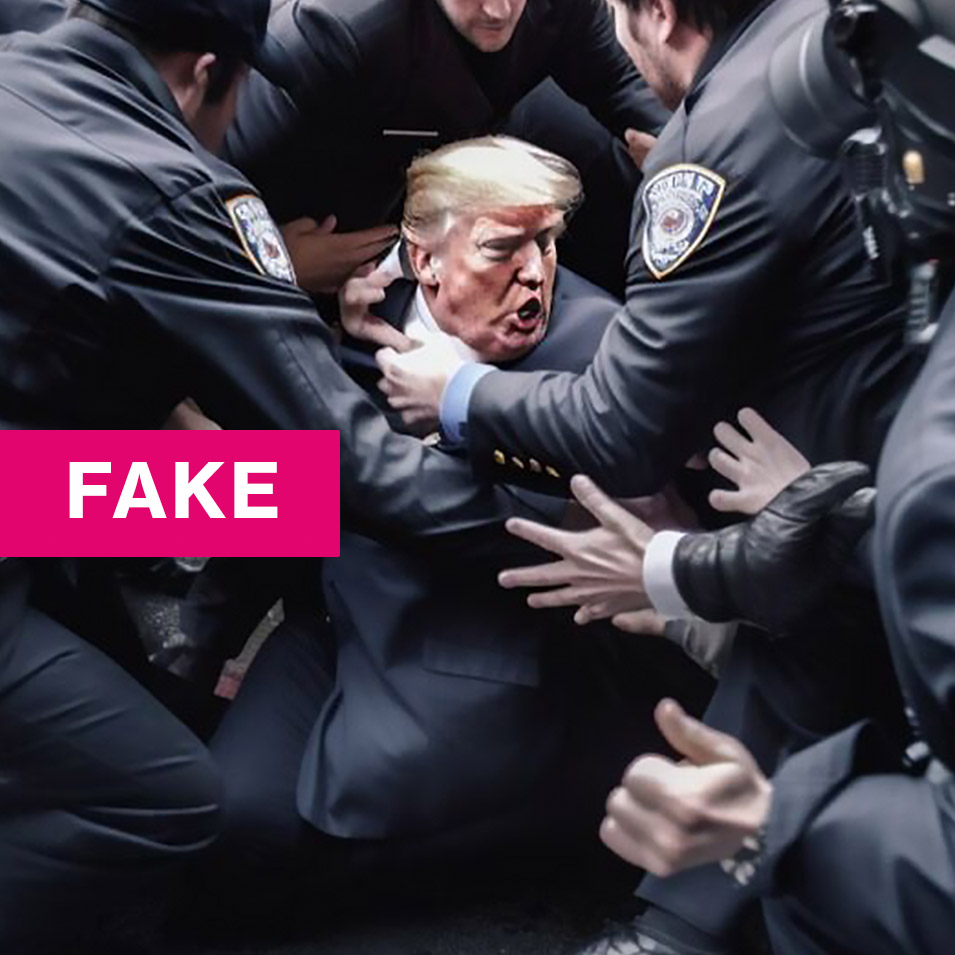
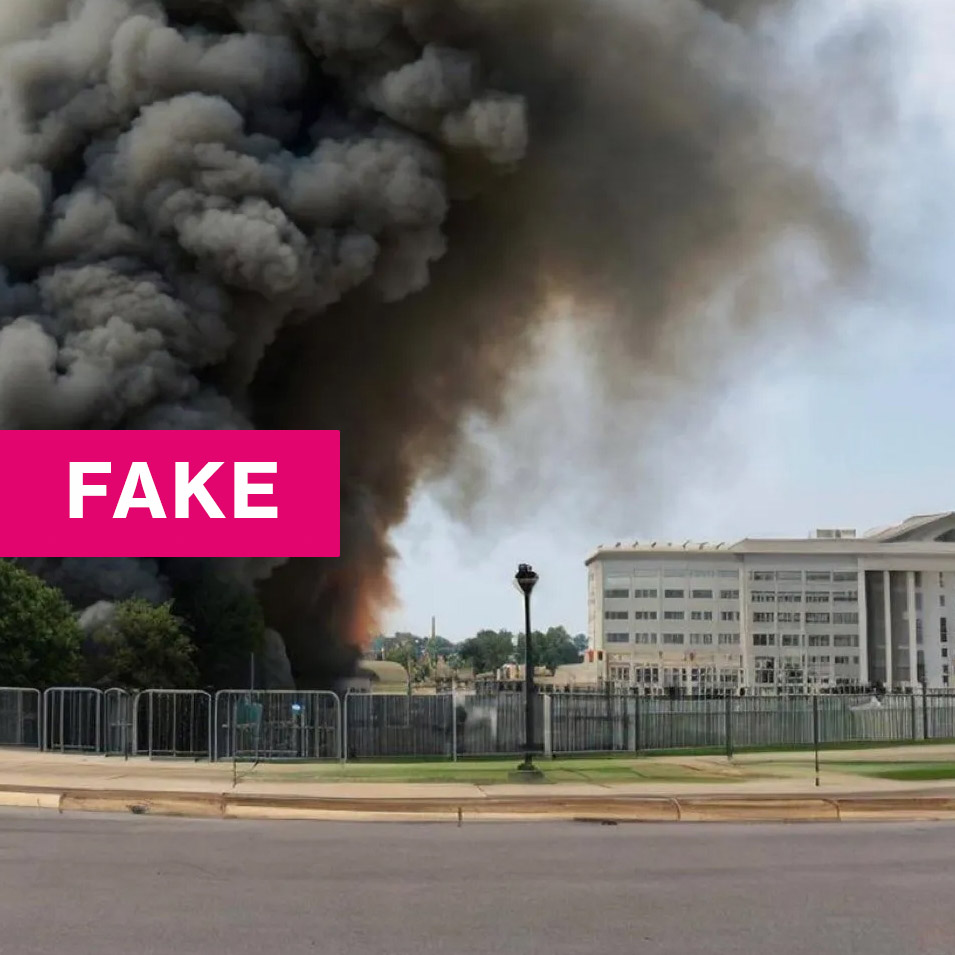
These are three of the most viral images of 2023. All fake; all seen and shared by millions of people.
The US has also said it will audit any AI that might pose threats to national security, including election interference. It’s a great step, says Benaich. But even the developers of these models don’t know their full capabilities: “The idea that governments or other independent bodies could force companies to fully test their models before they’re released seems unrealistic.”
Here’s the catch: it’s impossible to know all the ways a technology will be misused until it is used. “In 2023 there was a lot of discussion about slowing down the development of AI,” says Schaefer. “But we take the opposite view.”
Unless these tools get used by as many people in as many different ways as possible, we’re not going to make them better, he says: “We’re not going to understand the nuanced ways that these weird risks will manifest or what events will trigger them.”
Prediction: New forms of misuse will continue to surface as use ramps up. There will be a few standout examples, possibly involving electoral manipulation.
5
Will we come to grips with its costs?
The development costs of generative AI, both human and environmental, are also to be reckoned with. The invisible-worker problem is an open secret: we are spared the worst of what generative models can produce thanks in part to crowds of hidden (often poorly paid) laborers who tag training data and weed out toxic, sometimes traumatic, output during testing. These are the sweatshops of the data age.
In 2023, OpenAI’s use of workers in Kenya came under scrutiny by popular media outlets such as Time and the Wall Street Journal. OpenAI wanted to improve its generative models by building a filter that would hide hateful, obscene, and otherwise offensive content from users. But to do that it needed people to find and label a large number of examples of such toxic content so that its automatic filter could learn to spot them. OpenAI had hired the outsourcing firm Sama, which in turn is alleged to have used low-paid workers in Kenya who were given little support.
With generative AI now a mainstream concern, the human costs will come into sharper focus, putting pressure on companies building these models to address the labor conditions of workers around the world who are contracted to help improve their tech.
The other great cost, the amount of energy required to train large generative models, is set to climb before the situation gets better. In August, Nvidia announced Q2 2024 earnings of more than $13.5 billion, twice as much as the same period the year before. The bulk of that revenue ($10.3 billion) comes from data centers—in other words, other firms using Nvidia’s hardware to train AI models.
“The demand is pretty extraordinary,” says Nvidia CEO Jensen Huang. “We’re at liftoff for generative AI.” He acknowledges the energy problem and predicts that the boom could even drive a change in the type of computing hardware deployed. “The vast majority of the world’s computing infrastructure will have to be energy efficient,” he says.
Prediction: Greater public awareness of the labor and environmental costs of AI will put pressure on tech companies. But don’t expect significant improvement on either front soon.
6
Will doomerism continue to dominate policymaking?
Doomerism—the fear that the creation of smart machines could have disastrous, even apocalyptic consequences—has long been an undercurrent in AI. But peak hype, plus a high-profile announcement from AI pioneer Geoffrey Hinton in May that he was now scared of the tech he helped build, brought it to the surface.
Few issues in 2023 were as divisive. AI luminaries like Hinton and fellow Turing Award winner Yann LeCun, who founded Meta’s AI lab and who finds doomerism preposterous, engage in public spats, throwing shade at each other on social media.
Hinton, OpenAI CEO Sam Altman, and others have suggested that (future) AI systems should have safeguards similar to those used for nuclear weapons. Such talk gets people’s attention. But in an article he co-wrote in Vox in July, Matt Korda, project manager for the Nuclear Information Project at the Federation of American Scientists, decried these “muddled analogies” and the “calorie-free media panic” they provoke.
It’s hard to understand what’s real and what’s not because we don’t know the incentives of the people raising alarms, says Benaich: “It does seem bizarre that many people are getting extremely wealthy off the back of this stuff, and a lot of the people are the same ones who are mandating for greater control. It’s like, ‘Hey, I’ve invented something that’s really powerful! It has a lot of risks, but I have the antidote.’”
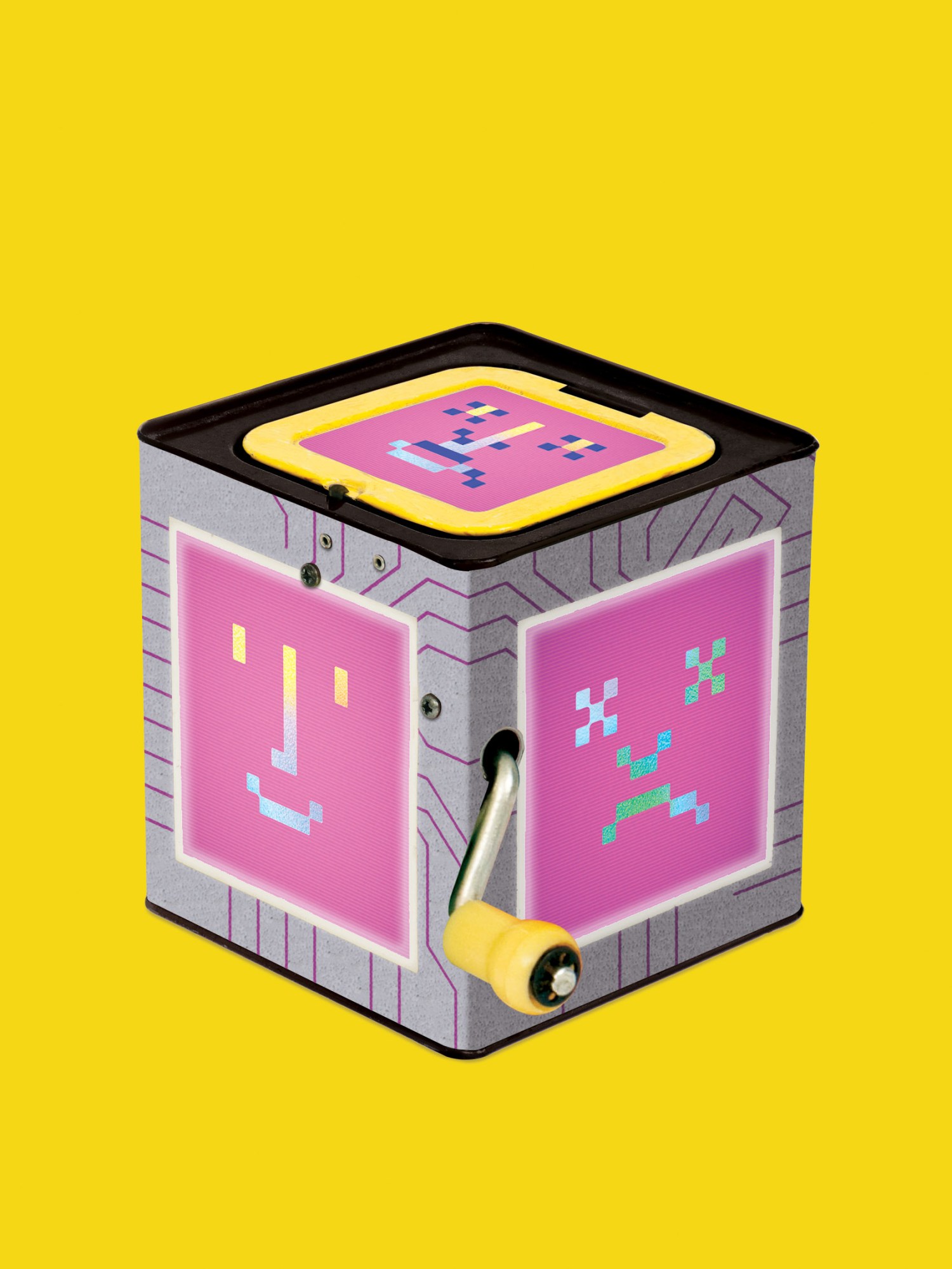
Some worry about the impact of all this fearmongering. On X, deep-learning pioneer Andrew Ng wrote: “My greatest fear for the future of AI is if overhyped risks (such as human extinction) lets tech lobbyists get enacted stifling regulations that suppress open-source and crush innovation.” The debate also channels resources and researchers away from more immediate risks, such as bias, job upheavals, and misinformation (see above).
“Some people push existential risk because they think it will benefit their own company,” says François Chollet, an influential AI researcher at Google. “Talking about existential risk both highlights how ethically aware and responsible you are and distracts from more realistic and pressing issues.”
Benaich points out that some of the people ringing the alarm with one hand are raising $100 million for their companies with the other. “You could say that doomerism is a fundraising strategy,” he says.
Prediction: The fearmongering will die down, but the influence on policymakers’ agendas may be felt for some time. Calls to refocus on more immediate harms will continue.
Still missing: AI’s killer app
It’s strange to think that ChatGPT almost didn’t happen. Before its launch in November 2022, Ilya Sutskever, cofounder and chief scientist at OpenAI, wasn’t impressed by its accuracy. Others in the company worried it wasn’t much of an advance. Under the hood, ChatGPT was more remix than revolution. It was driven by GPT-3.5, a large language model that OpenAI had developed several months earlier. But the chatbot rolled a handful of engaging tweaks—in particular, responses that were more conversational and more on point—into one accessible package. “It was capable and convenient,” says Sutskever. “It was the first time AI progress became visible to people outside of AI.”
The hype kicked off by ChatGPT hasn’t yet run its course. “AI is the only game in town,” says Sutskever. “It’s the biggest thing in tech, and tech is the biggest thing in the economy. And I think that we will continue to be surprised by what AI can do.”
But now that we’ve seen what AI can do, maybe the immediate question is what it’s for. OpenAI built this technology without a real use in mind. Here’s a thing, the researchers seemed to say when they released ChatGPT. Do what you want with it. Everyone has been scrambling to figure out what that is since.
“I find ChatGPT useful,” says Sutskever. “I use it quite regularly for all kinds of random things.” He says he uses it to look up certain words, or to help him express himself more clearly. Sometimes he uses it to look up facts (even though it’s not always factual). Other people at OpenAI use it for vacation planning (“What are the top three diving spots in the world?”) or coding tips or IT support.
Useful, but not game-changing. Most of those examples can be done with existing tools, like search. Meanwhile, staff inside Google are said to be having doubts about the usefulness of the company’s own chatbot, Bard (now powered by Google’s GPT-4 rival, Gemini, launched last month). “The biggest challenge I’m still thinking of: what are LLMs truly useful for, in terms of helpfulness?” Cathy Pearl, a user experience lead for Bard, wrote on Discord in August, according to Bloomberg. “Like really making a difference. TBD!”
Without a killer app, the “wow” effect ebbs away. Stats from the investment firm Sequoia Capital show that despite viral launches, AI apps like ChatGPT, Character.ai, and Lensa, which lets users create stylized (and sexist) avatars of themselves, lose users faster than existing popular services like YouTube and Instagram and TikTok.
“The laws of consumer tech still apply,” says Benaich. “There will be a lot of experimentation, a lot of things dead in the water after a couple of months of hype.”
Of course, the early days of the internet were also littered with false starts. Before it changed the world, the dot-com boom ended in bust. There’s always the chance that today’s generative AI will fizzle out and be eclipsed by the next big thing to come along.
Whatever happens, now that AI is fully in the mainstream, niche concerns have become everyone’s problem. As Schaefer says, “We’re going to be forced to grapple with these issues in ways that we haven’t before.”
Comments
Post a Comment